Retail Shake Academy
Data quality
Obtaining and managing data is a key part of a company’s decision-making process. It is therefore crucial to place special attention on the quality of the data chosen. At Retail Shake, we attach special importance to what we send to our clients. We also carry out several checks prior to sending.
My name is Sébastien Vanstavel, I am a data analyst at Retail Shake. I’m delighted to guide you through this lesson on data quality.
Data quality is defined by 5 pillars.
Accuracy
Often, the data selected by data-mining software does not comply with field realities. Even when it is very accurate, information can lose relevance due to the calculation method chosen by the solution. A poor-quality service will also tend to automatically include all recorded traffic, without previously sorting physical persons from robots for example. The resulting data is admittedly comprehensive but does not correspond to field realities.
To avoid this, use certified and approved Saas Software as a service. Retail Shake uses a data validation process to deliver reliable and comprehensive data to its clients.
Thoroughness
Another indispensable element for guaranteeing good quality data is thoroughness. Most decision-making errors are linked to data which is absent from the software or, at least, incomplete. The absence of certain data could be due to the choice of search filters (what’s known as the selector), which must be constantly updated, or by the unavailability of the data collection server. In order to guard against this, it can be useful to program regular filter validation tests in advance. A regular audit of the most important pages also enables you to check that the data search is optimized. In this way, data mining will be as comprehensive as possible.
Integrity
Data engineering should not be overlooked for obtaining valid and usable data. A formatting error during scraping can quickly make information simply unusable by your analysts. Bad signage is also the cause of many readability problems.
In order to guard against this, analysts should be free to modify the formatting of data themselves. They can thereby modify it as required and exploit it fully. It is also recommended to carry out regular data display tests on the interface to check that they remain compatible.
Freshness
Reactivity is key for a company’s strategic development. Taking decisions at the right time means having the most recent data possible. Unfortunately, technical problems can interrupt real-time data recovery. If no solution is found, it can take time to repair the fault. To fix this, companies must prepare a process to apply whenever a fault is noticed. It is also highly preferable to use a solution capable of transmitting your data in real-time via a monitoring table.
Coherence
It may seem beneficial to use several digital tools, either for competition monitoring or for gauging performance. But in reality, cross-checking several sources can quickly lead to data incoherences. By using a single solution for all of the company’s departments, data becomes, on the one hand, more coherent, and on the other, a lot more easily consultable by the various players. Similarly, drawing up a data management policy can standardize its use and quickly delete any data which is incoherent.
Each day at Retail Shake, one of my tasks is to ensure the quality of the data that we scrape. To this end, I use a number of tools enabling me, in particular, to track developments across our spiders, via monitoring dashboards (scrapydweb, grafana), or thanks to a log aggregation system (graylog). With tools such as these, in the case of an incident or non-compliance, I can find the source of the issue and notify the rest of the technical team so that they can implement any necessary corrective measures.
Thanks for watching this video and see you soon at the Retail Shake academy.
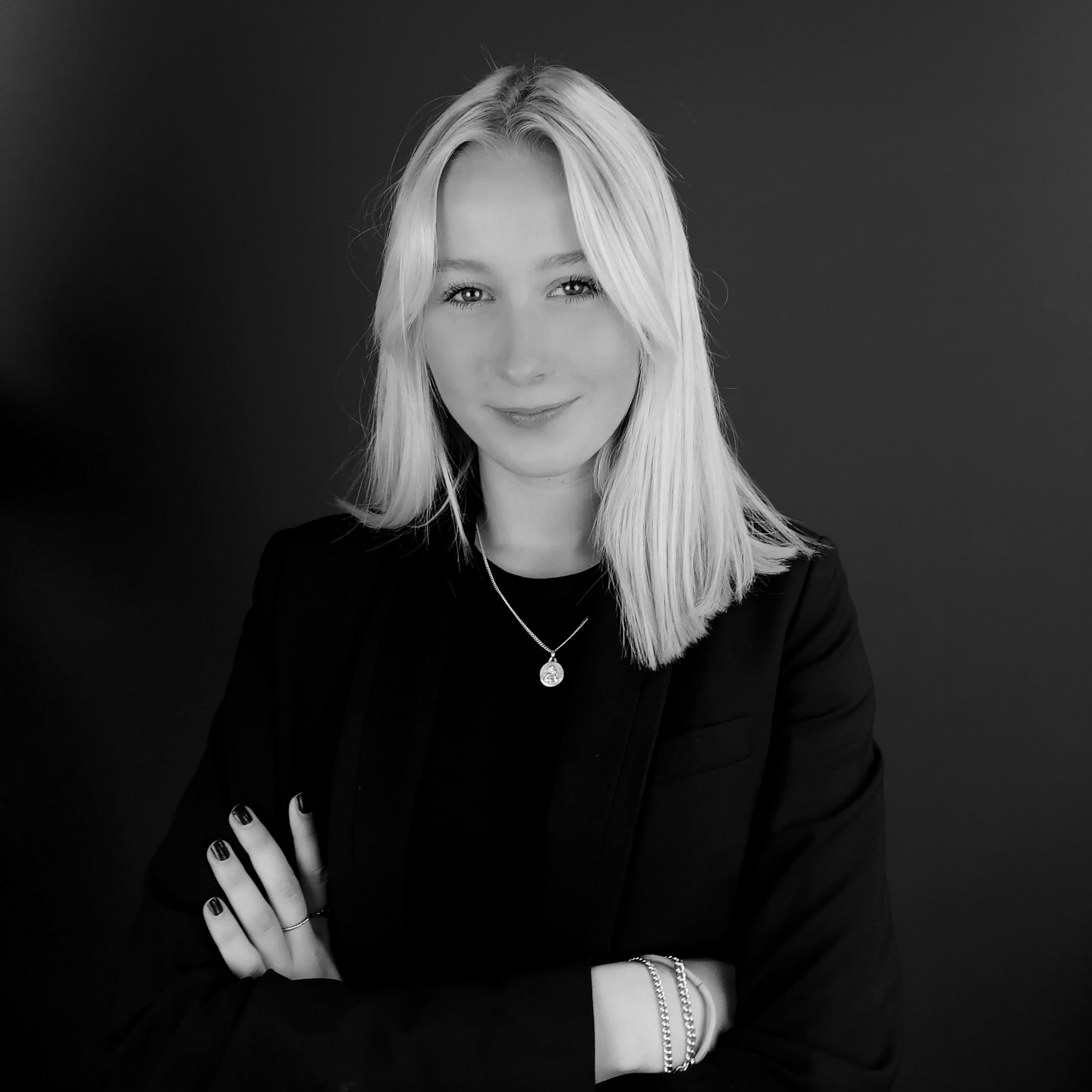
By Clémentine